Optimizing the utility of secondary outcomes in randomized controlled trials
Introduction
Randomized controlled trials (RCTs) are the cornerstone of evidence based medicine and are often considered the most important piece of evidence to guide clinical practice. To make sure RCTs are fit for their purpose, the design of such trials must be both statistically robust and clinically relevant. The sample size and statistical power of a study are mathematically related; it is widely accepted that the sample size should be sufficiently large to ensure a power of 80% or greater to avoid initiating a study that is destined to fail in rejecting the null hypothesis. In general, the predicted incidence of the primary outcome (in the control group) and the effect size (conferred by the test intervention) are the two most important elements that determine the mathematical relationship between sample size and the power of the study.
Secondary outcomes are common in RCTs. There are many reasons why researchers want to include a secondary outcome, including the interest to answer as many clinical questions as possible by doing only one trial—that is, as ‘niceties’. It is now a standard practice for researchers to predefine all secondary outcomes a priori in the trial’s protocol and also in the trial registry to avoid the temptation to conduct multiple post hoc analyses in an attempt to find a significant P value. What has not been thoroughly considered and widely adopted is how we can maximize the utility of secondary outcomes in RCTs.
The theoretical costs of secondary outcomes
Increasing the chance of a false positive result
Obviously, the more statistical tests that are conducted on the same dataset, the more likely researchers will obtain a test result showing a difference between the control and treatment groups, with a significant P value of <0.05, solely due to chance. Mathematically, the probability of obtaining a P value <0.05 is equal to 1−(1− α)n; where ‘α’ is the alpha (or P value, often taken as 0.05), and ‘n’ is the number of statistical tests conducted on the same study population. To overcome the potential of obtaining a falsely significant P value when there is truly none, some form of statistical correction is needed to make a valid statistical inference by avoiding the inflation of type I error. The Bonferroni adjustment (P value is significant only when the P value is ≤α/n), Tukey’s procedure (for post hoc pairwise comparisons in ANOVA), multiple permutation or bootstrap adjustment, or the Benjamini-Hochberg False Discovery Rate for genetic mutation analyses have been recommended to reduce the chance of obtaining a false positive result (1).
Misinterpreting secondary outcomes comparing two study groups with a P value >0.05 as they are equivalent or non-inferior to each other
Inexperienced researchers often consider secondary outcomes in a RCT as ‘niceties’ without giving too much thought about their utility, let alone how to improve their utility. As such, whether secondary outcomes are powered in a RCT is often not reported. This is not surprising because by ensuring secondary outcomes are adequately powered, the sample size needed can escalate substantially. Without being adequately powered, we would expect the secondary outcomes to be associated with a P value >0.05. One common misconception is that a lack of statistically significant differences in the secondary outcomes between two study groups in a superiority trial is concluded as the two groups are equivalent or non-inferior to each other (2). This is indeed far from true because ‘an absence of evidence’ is not the same as ‘evidence of absence’. The only conclusion for a statistically insignificant P value in a superiority trial is that we do not have evidence of superiority of one over the other (e.g., second red arrow from the top in Figure 1) which is different from concluding that there is non-inferiority between the two treatments. To conclude non-inferiority, a priori minimum difference in treatment effect has to be defined (by X in Figure 1) which is the smallest treatment effect size that is deemed clinically acceptable (2,3); and this would usually require a larger sample size than a superiority trial. Non-inferiority can only be concluded when the 95% confidence interval of the difference in treatment effects does not cross the a priori non-inferiority margin (as shown by the green arrow on the left in Figure 1).
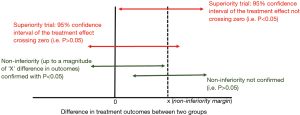
How to maximize the utility of secondary outcomes
Ideally, researchers should seriously power the secondary outcomes adequately (e.g., >80% after adjustment for multiple comparisons) and this would mean a power of ≥90% for the primary outcome is often needed (4). If the sample size is insufficient to achieve 80% power in rejecting the null hypotheses of the secondary outcomes, it would be better either not to include any secondary outcomes, or simply to choose alternative secondary outcomes that can be powered at 80% or more.
There are a few ways researchers can increase the power of a secondary outcome to improve its clinical utility. First, they can use a composite end-point to increase the incidence of the secondary outcome (provided the treatment under investigation does not move the different elements of the composite outcome in an opposite direction, e.g., hemorrhage and thrombosis for an anticoagulant trial). One common composite end-point in critical care is ventilator-free days or vasopressor-free days in which survival and the duration of ventilator or vasopressor dependency are pooled together. Ideally, all the elements constitute the composite end-point should have equal patient-centered significance; or else, the composite end-point may not be meaningful when it is primarily driven by the more frequent but clinically irrelevant events (5). Second, researchers can use an enrichment technique. Absolute risk reduction (ARR) between the intervention and control groups in a RCT can vary substantially dependent on baseline risk of the control group (6,7); whilst relative risk (RR) is usually a more stable attribute. As such, it is legitimate to analyze a subgroup of patients in a RCT who are most likely to experience the outcome under investigation as a secondary outcome, provided the risk is not so high that the outcome would occur regardless of whether the patients have received the study intervention (8). Third, we can predefine how to analyze the secondary outcomes with a more powerful statistical technique. For example, if cumulative incidence of events are high (due to the characteristics of the enrolled patients such as high risk cancer trials or use of a long follow up period with a low censoring rate) (https://sample-size.net/sample-size-survival-analysis/), survival analysis for time to event will be more efficient statistically than a Chi Square test or logistic regression (when the time factor is not fully utilized). As for survival analysis, use of flexible parametric survival models to estimate restricted mean survival time may also be more efficient when the proportional hazards assumption for the intervention under investigation is unlikely to be valid (9).
Finally, secondary outcomes would be useful to clinicians if researchers can at least define the magnitude of difference in secondary outcomes that would be deemed non-inferior to each other between the two treatment groups with the study sample size (Figure 1), even though such difference may not be as small as clinically acceptable in a typical non-inferiority trial (2,3). By doing so, researchers would give the results of a secondary outcome a quantitative clinical meaning beyond the numerical P values. Clinicians would then be able to conclude that any negative secondary outcome between two treatment groups would mean the two treatments are non-inferior to each other up to a certain non-inferiority margin.
Conclusions
Secondary or even tertiary outcomes are common in many RCTs, but often they are underpowered. Although some researchers argue that these are for exploratory purposes, the reality is that without statistical adjustment, false positives can easily occur and negative secondary outcomes are often misinterpreted as non-inferiority or equivalence between two treatment groups. The utility of secondary outcomes in a RCT can only be improved if researchers can consider them as important as the primary outcome when they design the trial.
Acknowledgments
Funding: None.
Footnote
Conflicts of Interest: Both authors have completed the ICMJE uniform disclosure form (available at http://dx.doi.org/10.21037/jeccm-20-136). KMH serves as an unpaid editorial board member of Journal of Emergency and Critical Care Medicine from May 2017 to April 2021. YH has no conflicts of interest to declare.
Ethical Statement: The authors are accountable for all aspects of the work in ensuring that questions related to the accuracy or integrity of any part of the work are appropriately investigated and resolved.
Open Access Statement: This is an Open Access article distributed in accordance with the Creative Commons Attribution-NonCommercial-NoDerivs 4.0 International License (CC BY-NC-ND 4.0), which permits the non-commercial replication and distribution of the article with the strict proviso that no changes or edits are made and the original work is properly cited (including links to both the formal publication through the relevant DOI and the license). See: https://creativecommons.org/licenses/by-nc-nd/4.0/.
References
- Noble WS. How does multiple testing correction work? Nat Biotechnol 2009;27:1135-7. [Crossref] [PubMed]
- Lesaffre E. Superiority, equivalence, and non-inferiority trials. Bull NYU Hosp Jt Dis 2008;66:150-4. [PubMed]
- Scott IA. Non-inferiority trials: determining whether alternative treatments are good enough. Med J Aust 2009;190:326-30. [Crossref] [PubMed]
- Jakobsen JC, Ovesen C, Winkel P, et al. Power estimations for non-primary outcomes in randomised clinical trials. BMJ Open 2019;9:e027092. [Crossref] [PubMed]
- Yehya N, Harhay MO, Curley MAQ, et al. Reappraisal of Ventilator-Free Days in Critical Care Research. Am J Respir Crit Care Med 2019;200:828-36. [Crossref] [PubMed]
- Ho KM. Importance of the baseline risk in determining sample size and power of a randomized controlled trial. J Emerg Crit Care Med 2018;2:70. [Crossref]
- Ho KM, Tan JA. Use of L'Abbé and pooled calibration plots to assess the relationship between severity of illness and effectiveness in studies of corticosteroids for severe sepsis. Br J Anaesth 2011;106:528-36. [Crossref] [PubMed]
- Ho KM, Holley A, Lipman J. Vena Cava filters in severely-injured patients: One size does not fit all. Anaesth Crit Care Pain Med 2019;38:305-7. [Crossref] [PubMed]
- Royston P, Parmar MK. Restricted mean survival time: an alternative to the hazard ratio for the design and analysis of randomized trials with a time-to-event outcome. BMC Med Res Methodol 2013;13:152. [Crossref] [PubMed]
Cite this article as: Harahsheh Y, Ho KM. Optimizing the utility of secondary outcomes in randomized controlled trials. J Emerg Crit Care Med 2021;5:8.