Mean platelet volume for prognostication after out-of-hospital cardiac arrest: a retrospective cohort analysis
Highlight box
Key findings
• For patients with out-of-hospital cardiac arrest (OHCA), the mean platelet volume (MPV) on the third day following admission was associated with in-hospital mortality.
What is known and what is new?
• The MPV serves as a biomarker for assessing the prognosis of several inflammatory conditions.
• The MPV may function as a prognostic indicator for mortality in individuals who have experienced OHCA.
What is the implication, and what should change now?
• The MPV has the potential to be used in a multimodal approach to the prognosis of patients with OHCA due to its low cost and widespread availability.
Introduction
Out-of-hospital cardiac arrest (OHCA) affects approximately 350,000 people in the US every year and is associated with rates of survival to and good functional status at hospital discharge of 10% and 8%, respectively (1). In Thailand, the average annual incidence of OHCA is 6,450 cases, with a discharge survival rate of 4.2% (2). The continued care of patients with OHCA should follow the six chains of survival, and post-cardiac arrest patients should receive multidisciplinary treatment in the intensive care unit (ICU) (3). A poor neurological outcome or brain death often leads to the withholding or withdrawal of treatment, including organ donation. Therefore, a multimodal approach should be adopted (4). The Pittsburgh Cardiac Arrest Category (PCAC) illness severity score is a simple, validated tool, that focuses on objective physical findings and is associated with survival to hospital discharge and a good functional outcome in patients with OHCA (5).
In the early post-return of spontaneous circulation (ROSC) phase, patients with OHCA may experience post-cardiac arrest syndrome (PCAS) (6), which is an inflammatory response that results from whole-body ischemia and reperfusion and involves the activation of platelet aggregation and modulation of the coagulation pathway (7). Platelets play crucial roles in blood clotting, inflammation, and the immune system. Measurement of the mean platelet volume (MPV) is one of the most widely used quantification methods in the complete blood count (CBC), and an increase in the MPV has been associated with mortality in various disease groups, such as critically ill patients with bloodstream infections, heart disease, and community-acquired pneumonia (8,9). However, the findings of previous studies on the MPV and patients with cardiac arrest have been inconclusive because of conflicting data on the predictive ability on the neurological outcomes and a lack of clear cutoff values. In one study, the MPV was not predictive of brain outcomes in comatose patients with OHCA who were treated with targeted temperature management (TTM) (10) whereas another study found an association between the MPV and clinical outcomes (11). Another study focused on platelet counts in patients with cardiac arrest receiving TTM (12).
To facilitate prognostication and appropriate allocation of treatment resources for this patient group, this study aimed to investigate the prognostic value of the MPV during the first 4 days of hospitalization in patients with OHCA. We present this article in accordance with the STROBE reporting checklist (available at https://jeccm.amegroups.com/article/view/10.21037/jeccm-23-150/rc).
Methods
Study design
This retrospective observational cohort study involved an analysis of data obtained from patients with ROSC after OHCA who were admitted to the medical ICU of Songklanagarind Hospital between January 2016 and December 2022. Adult patients (aged ≥18 years) whose CBC values were obtained on day 1 of hospitalization and at least once on any day between days 2 and 4 after admission were included. Patients with hematologic malignancies, immune thrombocytopenia, in-hospital cardiac arrest and an unknown discharge status were excluded. This study was conducted in accordance with the Declaration of Helsinki (as revised in 2013). This study was approved by the Ethics Committee of the Faculty of Medicine, Prince of Songkla University (REC 64-602-14-4) and the requirement for individual consent for this retrospective analysis was waived.
Setting
Post-cardiac arrest care was provided in the medical ICU at Songklanagarind Hospital, which is an 800-bed tertiary care center that includes a 10-bed medical ICU in southern Thailand. Five full-time intensivists and four cardiac interventionists were available 7 days a week. Patients with OHCA received treatment in accordance with the hospital policy guidelines. The patients were closely monitored in the medical ICU, and TTM at 33 ℃ was introduced for comatose patients, regardless of the initial cardiac rhythm, if there was no contraindication. However, the final decision for TTM was made by an on-duty ICU attending physician (13). Cardiac interventionists performed coronary angiography and percutaneous coronary intervention (PCI).
Neurological prognosis was evaluated 72 hours after either cardiac arrest or rewarming in patients who received TTM. The prognosis evaluation followed a multimodal approach that included neurological examination, electroencephalography and computed tomography of the brain. In non-survivors, a neurologist confirmed brain death.
Data collection
Data were collected from electronic medical records using a modified Utstein-style template that included baseline clinical characteristics such as sex, age, underlying diseases, cardiac arrest etiologies, time from cardiac arrest to sustained ROSC (which was defined as chest compression not being required for 20 minutes and signs of circulation persisting for at least 20 minutes) (14,15), cardiopulmonary resuscitation (CPR) time, first monitored rhythm, Acute Physiology and Chronic Health Evaluation (APACHE) II score in the first 24 hours of admission (16), Cerebral Performance Category (CPC) scale score at hospital discharge (14), and CBC on days 1–4 of hospitalization. Day 1 was defined as the interval from admission to 5:00 AM the next day; all other days were calendar days that were considered from 5:00 AM on the respective day to 4.59 AM the following day.
Based on the results of a standardized physical examination conducted by a doctor within 6 hours after ROSC, as previously described (5,17), we retroactively assigned PCAC ratings. The Full Outline of Unresponsiveness brainstem (18) and motor subscores as well as the Serial Organ Failure Assessment cardiac and respiratory subscales were used to calculate the PCAC score (19). The four PCAC levels were awake, comatose with mild cardiopulmonary dysfunction, comatose with moderate-to-severe cardiopulmonary dysfunction, and comatose with at least one absent brainstem reflex.
Venous samples were collected in tubes containing ethylenediaminetetraacetic acid. The CBC, including MPV, was determined using an automated hematology analyzer (XT-1800i, Sysmex Corporation, Tokyo, Japan), which automatically ascertained the MPV within 30 minutes of sample collection. MPV1 was defined as the MPV on day 1 of ICU admission. MPV2, MPV3, and MPV4 were defined as the MPV on days 2, 3, and 4, respectively, after ICU admission. If multiple CBCs were obtained on a given day, the earliest CBC result was used.
Primary outcomes
The primary outcome was in-hospital mortality. The secondary outcome was poor neurological outcome at hospital discharge, which was assessed using the CPC, rated on a scale of 3–5, where CPC 3 indicates severe cerebral disability (conscious but disabled and dependent), CPC 4 denotes a comatose/vegetative state (unconscious) and CPC 5 indicates brain death (certified brain death or death by traditional criteria).
Statistical analysis
Assuming an area under the curve (AUC) of 0.7 with marginal error of 0.07 and 95% confidence interval (CI), the required sample size was 114 (20). Continuous variables are presented as means with standard deviations or medians with interquartile ranges (IQRs), depending on the distribution of the data. Discrete variables are presented as numbers and percentages. Student’s t-test or the Wilcoxon rank-sum test was used to compare the differences between continuous variables whereas Pearson’s chi-square or Fisher’s exact test was used to compare the differences between discrete variables. On days 2–4, some MPV data were absent given that we did not routinely measure MPV every day or patients had died during that period. However, the statistical analysis did not include imputations for missing data.
To analyze the change in the MPV over 4 days between patients with poor and good neurological outcomes, we used a generalized estimating equation (GEE). Logistic regression models were used to identify factors that affected the outcomes. First, we used univariable logistic regression models for MPV, severity score, and other selected clinical parameters with a significance threshold of 0.2, and then created two different multivariable logistic regression models to avoid collinearity. Model 1 was adjusted for the APACHE II score, while Model 2 was adjusted for the presence of diabetes mellitus, hypertension, chronic kidney disease, CPR time, shockable rhythm, PCI, and PCAC score. Additionally, the ability of MPV to predict poor neurological outcomes was assessed by calculating the AUC, using cutoff points to determine the sensitivity and specificity with 95% CIs as well as by calculating the positive and negative likelihood ratios. By using the respective cutoff points from the AUC, threshold values with a specificity of 90% were then determined for each marker (21). Given its greater utility in predicting in-hospital mortality and poor neurological outcomes, as opposed to ruling these out in the post-cardiac arrest setting, a high specificity cutoff was used. A test was considered highly, moderately, and poorly accurate if the AUCs were ≥0.9, ≥0.7 but <0.9, and >0.5 but <0.7, respectively; a value ≤0.5 indicated coincidental results (22). Due to unbalanced and non-random samples, we compared the AUCs between two variables using the bootstrap-corrected estimator of AUC (23). Internal validation was performed using a bootstrapping resampling procedure with 1,000 replicates to evaluate the accuracy of the model.
Statistical significance was set at P<0.05 all comparisons. All statistical analyses were performed using STATA version 16 (StataCorp LP, College Station, TX, USA) and R software version 4.2.2 (R Foundation for Statistical Computing, Vienna, Austria).
Results
Patient flow and recruitment
All 231 patients with OHCA and ROSC who were admitted to the medical ICU were screened for this study: 84 were excluded and 147 patients were enrolled. Among them, these 110 patients (74.8%) were defined as having poor neurological outcomes, and 82 patients (55.8%) died (Figure 1). The overall median hospital length of stay was 9 days (IQR, 3 to 21 days).
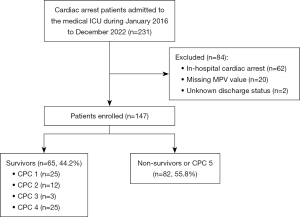
Patient characteristics
The baseline characteristics of all patients were stratified into four groups: survivors or non-survivors and good or poor neurological outcomes at hospital discharge (Table 1).
Table 1
Variables | Total (n=147) | Mortality | Neurological outcomes | |||||
---|---|---|---|---|---|---|---|---|
Survival (n=65) | Death (n=82) | P | Good (n=37) | Poor (n=110) | P | |||
Age (years) | 60 [52–73] | 57 [51–68] | 64 [55–76] | 0.02 | 57 [51–68] | 64 [55–76] | 0.01 | |
Male | 106 (72.1) | 45 (69.2) | 61 (74.4) | 0.48 | 28 (75.7) | 78 (70.9) | 0.57 | |
History | ||||||||
Diabetes mellitus | 34 (23.1) | 6 (9.2) | 28 (34.1) | <0.001 | 3 (8.1) | 31 (28.2) | 0.01 | |
Hypertension | 64 (43.5) | 21 (32.3) | 43 (52.4) | 0.01 | 11 (29.7) | 53 (48.2) | 0.050 | |
Obstructive lung | 16 (10.9) | 8 (12.3) | 8 (9.8) | 0.62 | 3 (8.1) | 13 (11.8) | 0.76 | |
Malignancy | 6 (4.1) | 4 (6.2) | 2 (2.4) | 0.40 | 2 (5.4) | 4 (3.6) | 0.64 | |
CAD | 23 (15.6) | 6 (9.2) | 17 (20.7) | 0.50 | 5 (13.5) | 18 (16.4) | 0.68 | |
Stroke | 7 (4.8) | 4 (6.2) | 3 (3.7) | 0.70 | 2 (5.4) | 5 (4.5) | >0.99 | |
CKD | 27 (18.4) | 6 (9.2) | 21 (25.6) | 0.01 | 2 (5.4) | 25 (22.7) | 0.01 | |
Characteristics of cardiac arrest | ||||||||
Witnessed arrest | 117 (79.6) | 55 (84.6) | 62 (75.6) | 0.17 | 32 (86.5) | 85 (77.3) | 0.22 | |
Bystander CPR | 51 (34.7) | 23 (35.2) | 28 (34.1) | 0.87 | 17 (45.9) | 34 (30.9) | 0.09 | |
Time from cardiac arrest to sustained ROSC (minutes) | 30 [14–40] | 21 [10–35] | 31 [17–44] | 0.01 | 17 [7–33] | 30 [17–43] | 0.005 | |
CPR time (minutes) | 11 [7–21] | 9 [6–18] | 15 [8–26] | 0.007 | 7 [4–18] | 14 [8–22] | 0.003 | |
First monitored rhythm | ||||||||
Shockable | 53 (36.1) | 30 (46.2) | 23 (28.0) | 0.02 | 21 (56.8) | 32 (29.1) | 0.002 | |
VF | 51 (34.7) | 28 (43.1) | 23 (28.0) | 0.054 | 19 (51.4) | 32 (29.1) | 0.01 | |
VT | 2 (1.4) | 2 (3.1) | 0 | 0.19 | 2 (5.4) | 0 | 0.06 | |
Non-shockable | 94 (63.9) | 35 (53.8) | 59 (72.0) | 0.02 | 16 (43.2) | 78 (70.9) | 0.002 | |
Asystole | 37 (25.2) | 14 (21.5) | 23 (28.0) | 0.36 | 6 (16.2) | 31 (28.2) | 0.14 | |
PEA | 57 (38.8) | 21 (32.3) | 36 (43.9) | 0.15 | 10 (27.0) | 47 (42.7) | 0.09 | |
Causes of arrest | ||||||||
Cardiac | 91 (61.9) | 42 (64.6) | 49 (59.8) | 0.54 | 28 (75.7) | 63 (57.3) | 0.046 | |
STEMI | 40 (27.2) | 21 (32.3) | 19 (23.2) | 0.21 | 16 (43.2) | 24 (21.8) | 0.01 | |
NSTEMI | 35 (23.8) | 11 (16.9) | 24 (29.3) | 0.08 | 6 (16.2) | 29 (26.4) | 0.21 | |
Non-MI | 16 (10.9) | 10 (15.4) | 6 (7.3) | 0.11 | 6 (16.2) | 10 (9.1) | 0.23 | |
Trauma | 2 (1.4) | 1 (1.5) | 1(1.2) | >0.99 | 0 | 2 (1.8) | >0.99 | |
Respiratory | 37 (25.2) | 16 (24.6) | 21 (25.6) | 0.89 | 7 (18.9) | 30 (27.3) | 0.31 | |
Metabolic | 10 (6.8) | 3 (4.6) | 7 (8.5) | 0.51 | 2 (5.4) | 8 (7.3) | >0.99 | |
Unidentified | 4 (2.7) | 2 (3.1) | 2 (2.4) | >0.99 | 0 | 4 (3.6) | 0.57 | |
Severity and procedures undertaken at hospital arrival | ||||||||
APACHE II score | 25 [20–28] | 22 [17–26] | 26 [23–30] | <0.001 | 22 [17–26] | 26 [23–30] | <0.001 | |
PCAC score | 2 [2–4] | 2 [1–3] | 3 [2–4] | <0.001 | 2 [1–3] | 3 [2–4] | <0.001 | |
PCI performed | 37 (25.2) | 22 (33.8) | 15 (18.3) | 0.03 | 18 (48.6) | 19 (17.3) | <0.001 | |
TTM | 80 (54.4) | 33 (50.8) | 47 (57.3) | 0.42 | 14 (37.8) | 66 (60.0) | 0.01 |
Data are presented as median [interquartile range] or n (%). CAD, coronary artery disease; CKD, chronic kidney disease; CPR, cardiopulmonary resuscitation; ROSC, return of spontaneous circulation; VF, ventricular fibrillation; VT, ventricular tachycardia; PEA, pulseless electrical activity; STEMI, ST-elevation myocardial infarction; NSTEMI, non-ST-elevation myocardial infarction; MI, myocardial infarction; APACHE II, Acute Physiology and Chronic Health Evaluation II; PCAC, Pittsburgh Cardiac Arrest Category; PCI, percutaneous coronary intervention; TTM, targeted temperature management.
Non-survivors were older (64 vs. 57 years, P=0.02) and more likely to have a history of diabetes mellitus (34.1% vs. 9.2%, P<0.001), hypertension (52.4% vs. 32.3%, P=0.01), and chronic kidney disease (25.6% vs. 9.2%, P=0.01) than survivors. Compared with survivors, non-survivors experienced a longer time from cardiac arrest to sustained ROSC (31 vs. 21 minutes, P=0.01), a longer CPR time (15 vs. 9 minutes, P=0.007), had more severe disease (APACHE II score 26 vs. 22, P<0.001), and had higher PCAC scores (3 vs. 2, P<0.001). Conversely, survivors were more likely to have an initial shockable rhythm (46.2% vs. 28.0%, P=0.02) and undergo PCI (33.8% vs. 18.3%, P=0.03).
MPV results and predictors of outcomes
The MPV in the first 3 days was significantly correlated with in-hospital mortality but not with neurological outcomes. Based on the GEE analysis with independent correlation, we found that 4 days after admission, the MPV was higher in non-survivors than in survivors (P<0.001; Table 2 and Figure 2).
Table 2
MPV (fL) | N | Mortality | Neurological outcomes | |||||
---|---|---|---|---|---|---|---|---|
Survival | Death | P (<0.001*) | Good | Poor | P (0.10*) | |||
MPV 1 | 147 | 10.3 [9.6–10.8] | 10.6 [9.8–11.4] | 0.01 | 10.3 [9.6–10.8] | 10.5 [9.8–11.2] | 0.22 | |
MPV 2 | 135 | 10.2 [9.5–11.0] | 10.6 [10.0–11.3] | 0.01 | 10.4 [9.4–10.9] | 10.4 [9.9–11.3] | 0.25 | |
MPV 3 | 92 | 10.4 [9.4–11.3] | 11.0 [10.6–11.9] | 0.001 | 10.4 [9.5–11.4] | 11.0 [10.3–11.6] | 0.17 | |
MPV 4 | 89 | 11.2 [10.6–11.7] | 11.3 [10.6–11.9] | 0.52 | 11.2 [10.8–11.7] | 11.3 [10.6–11.8] | 0.60 |
Data are presented as median [interquartile range]. *, based on generalized estimation equation analysis (independent correlation). MPV, mean platelet volume.
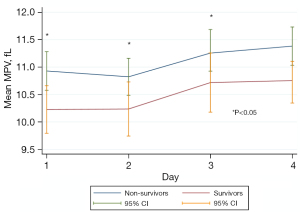
Variables that affected in-hospital mortality were introduced in the univariable analysis (Table 3). Due to age being included in the APACHE II score and CPR time being involved in the time from cardiac arrest to sustained ROSC, we selected the APACHE II score, CPR time and other variables for the multivariable logistic regression. After checking for collinearity, the APACHE II score needed to be separated and analyzed in Model 1, while other variables were analyzed in Model 2.
Table 3
Variables | OR (95% CI) | P value |
---|---|---|
Age | 1.02 (0.99–1.04) | 0.055 |
Diabetes mellitus | 5.09 (1.96–13.26) | 0.001 |
Hypertension | 2.31 (1.17–4.54) | 0.01 |
Chronic kidney disease | 3.38 (1.27–8.98) | 0.01 |
Time from cardiac arrest to sustained ROSC | 1.02 (1.00–1.04) | 0.01 |
CPR time | 1.04 (1.01–1.08) | 0.01 |
Shockable rhythm | 0.45 (0.23–0.90) | 0.02 |
APACHE II score | 1.18 (1.11–1.28) | <0.001 |
PCAC score | 2.13 (1.52–2.98) | <0.001 |
PCI | 0.44 (0.20–0.93) | 0.03 |
OR, odds ratio; CI, confidence interval; ROSC, return of spontaneous circulation; CPR, cardiopulmonary resuscitation; APACHE II, Acute Physiology and Chronic Health Evaluation II; PCAC, Pittsburgh Cardiac Arrest Category; PCI, percutaneous coronary intervention.
The results of multivariable analysis are demonstrated in Table 4. In Model 1, when adjusted for the APACHE II score, the MPVs on the first 3 days were associated with mortality. In Model 2, when adjusted for diabetes mellitus, hypertension, chronic kidney disease, CPR time, shockable rhythm, PCI performed, and PCAC score, only the MPVs on days 2 and 3 were associated with mortality.
Table 4
Parameters | Model 1* | Model 2** | |||
---|---|---|---|---|---|
Adjusted OR (95% CI) | P value | Adjusted OR (95% CI) | P value | ||
MPV 1 | 1.47 (1.01–2.14) | 0.043 | 1.53 (0.99–2.36) | 0.051 | |
MPV 2 | 1.49 (1.01–2.22) | 0.044 | 1.74 (1.08–2.81) | 0.02 | |
MPV 3 | 1.92 (1.21–3.06) | 0.006 | 2.50 (1.39–4.47) | 0.002 | |
MPV 4 | 1.27 (0.78–2.09) | 0.33 | 1.52 (0.86–2.68) | 0.14 |
*, adjusted for APACHE II score; **, adjusted for diabetes mellitus, hypertension, chronic kidney disease, CPR time, shockable rhythm, PCI performed, and PCAC. MPV, mean platelet volume; OR, odds ratio; CI, confidence interval; APACHE II, Acute Physiology and Chronic Health Evaluation II; CPR, cardiopulmonary resuscitation; PCI, percutaneous coronary intervention; PCAC, Pittsburgh Cardiac Arrest Category.
The MPVs on days 3 and 2 had accuracy in predicting in-hospital with AUCs of 0.709 (95% CI: 0.599–0.818) and 0.609 (95% CI: 0.512–0.705), respectively; the difference in AUCs were not significant (P=0.51).
We selected the MPV on day 3 as a surrogate prognostic marker since the timeframe is in line with the current guidelines that recommend performing prognostication no earlier than 72 hours after ROSC in comatose patients (4). We performed internal validation of MPV 3 via non-parametric receiver operating characteristic curve analysis with 1,000 bootstrap samples, and the results showed acceptable predictive performance (AUC =0.693, 95% CI: 0.584–0.793). We found that the optimal cutoff of MPV on day 3 was ≥11.7 fL with a sensitivity of 30.36% and specificity of 94.44% (Table 5).
Table 5
Cutoff point | Sensitivity (%) | Specificity (%) | Correctly classified (%) | LR+ | LR− |
---|---|---|---|---|---|
≥11.7 fL | 30.36 | 94.44 | 55.43 | 5.46 | 0.74 |
≥11.9 fL | 26.79 | 94.44 | 53.26 | 4.82 | 0.77 |
≥12 fL | 21.43 | 94.44 | 50.00 | 3.86 | 0.83 |
MPV, mean platelet volume; LR+, positive likelihood ratio; LR−, negative likelihood ratio.
On comparing the accuracy of predicting mortality with the well-known APACHE II and PCAC scores, the APACHE II score showed the highest AUC, followed by the MPV on day 3 and the PCAC score, albeit without any significant difference among the three scores (AUC =0.750, 95% CI: 0.649–0.851; AUC =0.709, 95% CI: 0.599–0.818; and AUC =0.703, 95% CI: 0.596–0.811, respectively; Figure 3).
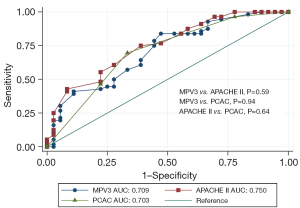
To determine the added diagnostic ability of MPV on day 3, we combined the APACHE II score with the MPV on day 3 (AUC =0.773, 95% CI: 0.676–0.869). At a cut-off point of 41.4, the sensitivity was 33.93% and the specificity was 97.22%.
Discussion
In this retrospective analysis, the MPVs on days 2 and 3 of ICU admission were associated with in-hospital mortality after adjusting for confounding factors. As a predictor of mortality, the MPV on day 3 was comparable to that on day 2. For timing purposes and its moderate accuracy in predicting in-hospital mortality in patients with OHCA, the MPV on day 3 should be considered a prognostic tool. However, it is unable to predict poor neurological outcomes.
The MPV has been used as a relatively novel marker for inflammation-related diseases, such as myocardial infarction or sepsis. It reflects the average size of platelets, which increases under inflammatory or thrombotic conditions. In PCAS, the mechanism is similar to that of sepsis, with inflammatory cytokine activation resulting in an increase in MPV.
MPV kinetics in septic shock were investigated by Vandon-Bounes et al. (24), wherein a 10-day MPV >11.6 fL was an independent predictor of 90-day mortality. The authors raised concerns about an increase in the MPV during sepsis without a return to the initial value, which is linked to more severe illness. PCAS was proposed as a sepsis-like syndrome in which abnormalities after cardiac arrest mimic the immunological and coagulation disorders observed in severe sepsis (25). Based on the available data, MPV kinetics in patients with OHCA were studied for a maximum of 96 hours after admission. Based on the mechanism of septic shock, we suggest that MPV should be monitored over a longer period after cardiac arrest.
In our study, the MPV on day 3 of admission differed between survivors and non-survivors and was significantly associated with in-hospital mortality after adjusting for severity and other variables. The wide 95% CI reflects the small sample size, which makes it difficult to draw strong conclusions regarding the prognostic validity of the MPV.
Our data showed that, as a predictor of in-hospital mortality, the highest accuracy was determined for the MPV on day 3 or 48 hours after cardiac arrest. A retrospective study in Korea by Chung et al. (11) revealed that the MPV at admission could independently predict 30-day mortality and a 30-day poor neurological outcome. However, a retrospective study by Cotoia et al. (10) in Belgium showed that the MPV was not associated with the 3-month neurological outcome in patients with OHCA. These conflicting results can be attributed to several factors. First, the difference in baseline characteristics of the patients with OHCA between our cohort and those in previous studies may have affected mortality. We had a small number of bystander CPR procedures, similar to that in the study by Chung et al. (35.8% vs. 33.1% in the study by Chung et al. vs. 66% in the study by Cotoia et al.), and a lower shockable presentation rhythm (34.3% vs. 29.8% in the study by Chung et al. vs. 39% in the study by Cotoia et al.), which resulted in a low chance of survival. Second, in the study by Cotoia et al., the authors selected only comatose patients with OHCA who received TTM, compared with our cohort wherein patients who received TTM comprised only 54.4% of the cases. Third, our patients had overall median hospital length of stay of 9 days. The primary outcomes of in-hospital mortality and a poor CPC score at hospital discharge were shorter than those in the two previously mentioned studies, which were 30-day mortality and 3-month neurological outcome.
The ability of the MPV to predict mortality on day 3 was comparable to the well-known APACHE II and PCAC scores. However, the APACHE II system combines 12 complex physiological variables with various sources of error and overestimation (26). Therefore, the MPV on day 3 and the PCAC are simpler and easier tools that can be used even in resource-limited situations. Nevertheless, our findings indicated that MPV on day 3 could be incorporated into the APACHE II scores for greater accuracy. The timing of the MPV obtained on day 3 is in line with the guidelines that recommend prognostication be reserved until at least 72 hours after ROSC. Based on the current guidelines, we encourage the use of a multimodal approach to improve the neurological prognosis of patients with OHCA.
Our study has certain limitations. First, it was a single-center retrospective study, which implies the possibility of selection bias. Second, we only selected patients with MPV data on admission and at least two MPV data point in the first 4 days after hospitalization. Third, per our post-arrest care protocol, C-reactive protein (CRP) and interleukin-6 (IL-6) were not routinely measured; therefore, we did not compare MPV with other inflammatory markers, such as CRP or IL-6, which are exact indicators. Fourth, we did not include all confounding factors that might affect MPV values, such as thyroid dysfunction, obesity, and smoking (27). We also did not exclude patients in whom the cause of arrest was infection or sepsis, nor patients taking antiplatelet agents which may alter the MPV. Fifth, we analyzed the outcomes separately without adjusting for multiple comparisons. Finally, we focused on in-hospital mortality and neurological outcomes at discharge. Long-term mortality or neurological outcomes were not assessed. However, the CPC score at discharge constitutes a useful surrogate measure of long-term survival in patients (28).
Conclusions
In patients with OHCA, there was an independent association between the MPV on day 3 after admission and in-hospital mortality. Larger studies are required to confirm these findings and explore the role of MPV in predicting neurological outcomes.
Acknowledgments
Funding: None.
Footnote
Reporting Checklist: The authors have completed the STROBE reporting checklist. Available at https://jeccm.amegroups.com/article/view/10.21037/jeccm-23-150/rc
Data Sharing Statement: Available at https://jeccm.amegroups.com/article/view/10.21037/jeccm-23-150/dss
Peer Review File: Available at https://jeccm.amegroups.com/article/view/10.21037/jeccm-23-150/prf
Conflicts of Interest: Both authors have completed the ICMJE uniform disclosure form (available at https://jeccm.amegroups.com/article/view/10.21037/jeccm-23-150/coif). The authors have no conflicts of interest to declare.
Ethical Statement: The authors are accountable for all aspects of the work in ensuring that questions related to the accuracy or integrity of any part of the work are appropriately investigated and resolved. This study was conducted in accordance with the Declaration of Helsinki (as revised in 2013). This study was approved by the Ethics Committee of the Faculty of Medicine, Prince of Songkla University (REC 64-602-14-4) and the requirement for individual consent for this retrospective analysis was waived.
Open Access Statement: This is an Open Access article distributed in accordance with the Creative Commons Attribution-NonCommercial-NoDerivs 4.0 International License (CC BY-NC-ND 4.0), which permits the non-commercial replication and distribution of the article with the strict proviso that no changes or edits are made and the original work is properly cited (including links to both the formal publication through the relevant DOI and the license). See: https://creativecommons.org/licenses/by-nc-nd/4.0/.
References
- Virani SS, Alonso A, Benjamin EJ, et al. Heart Disease and Stroke Statistics-2020 Update: A Report From the American Heart Association. Circulation 2020;141:e139-596. [Crossref] [PubMed]
- Huabbangyang T, Klaiangthong R, Silakoon A, et al. The comparison of emergency medical service responses to and outcomes of out-of-hospital cardiac arrest before and during the COVID-19 pandemic in Thailand: a cross-sectional study. Int J Emerg Med 2023;16:9. [Crossref] [PubMed]
- Panchal AR, Bartos JA, Cabañas JG, et al. Part 3: Adult Basic and Advanced Life Support: 2020 American Heart Association Guidelines for Cardiopulmonary Resuscitation and Emergency Cardiovascular Care. Circulation 2020;142:S366-468. [Crossref] [PubMed]
- Nolan JP, Sandroni C, Böttiger BW, et al. European Resuscitation Council and European Society of Intensive Care Medicine guidelines 2021: post-resuscitation care. Intensive Care Med 2021;47:369-421. [Crossref] [PubMed]
- Coppler PJ, Elmer J, Calderon L, et al. Validation of the Pittsburgh Cardiac Arrest Category illness severity score. Resuscitation 2015;89:86-92. [Crossref] [PubMed]
- Neumar RW, Nolan JP, Adrie C, et al. Post-cardiac arrest syndrome: epidemiology, pathophysiology, treatment, and prognostication. A consensus statement from the International Liaison Committee on Resuscitation (American Heart Association, Australian and New Zealand Council on Resuscitation, European Resuscitation Council, Heart and Stroke Foundation of Canada, InterAmerican Heart Foundation, Resuscitation Council of Asia, and the Resuscitation Council of Southern Africa); the American Heart Association Emergency Cardiovascular Care Committee; the Council on Cardiovascular Surgery and Anesthesia; the Council on Cardiopulmonary, Perioperative, and Critical Care; the Council on Clinical Cardiology; and the Stroke Council. Circulation 2008;118:2452-83. [Crossref] [PubMed]
- Wada T. Coagulofibrinolytic Changes in Patients with Post-cardiac Arrest Syndrome. Front Med (Lausanne) 2017;4:156. [Crossref] [PubMed]
- Korniluk A, Koper-Lenkiewicz OM, Kamińska J, et al. Mean Platelet Volume (MPV): New Perspectives for an Old Marker in the Course and Prognosis of Inflammatory Conditions. Mediators Inflamm 2019;2019:9213074. [Crossref] [PubMed]
- Reangvilaikul T, Udompongpaiboon P, Vattanavanit V. Predicting acute respiratory distress syndrome in influenza pneumonia patients using delta mean platelet volume. BMC Pulm Med 2021;21:405. [Crossref] [PubMed]
- Cotoia A, Franchi F, De Fazio C, et al. Platelet indices and outcome after cardiac arrest. BMC Emerg Med 2018;18:31. [Crossref] [PubMed]
- Chung SP, Yune HY, Park YS, et al. Usefulness of mean platelet volume as a marker for clinical outcomes after out-of-hospital cardiac arrest: a retrospective cohort study. J Thromb Haemost 2016;14:2036-44. [Crossref] [PubMed]
- Kim HJ, Park KN, Kim SH, et al. Time course of platelet counts in relation to the neurologic outcome in patients undergoing targeted temperature management after cardiac arrest. Resuscitation 2019;140:113-9. [Crossref] [PubMed]
- Vattanavanit V, Uppanisakorn S, Nilmoje T. Post out-of-hospital cardiac arrest care in a tertiary care center in southern Thailand: from emergency department to intensive care unit. Hong Kong Journal of Emergency Medicine 2020;27:155-61. [Crossref]
- Jacobs I, Nadkarni V, Bahr J, et al. Cardiac arrest and cardiopulmonary resuscitation outcome reports: update and simplification of the Utstein templates for resuscitation registries: a statement for healthcare professionals from a task force of the International Liaison Committee on Resuscitation (American Heart Association, European Resuscitation Council, Australian Resuscitation Council, New Zealand Resuscitation Council, Heart and Stroke Foundation of Canada, InterAmerican Heart Foundation, Resuscitation Councils of Southern Africa). Circulation 2004;110:3385-97. [Crossref] [PubMed]
- Dankiewicz J, Cronberg T, Lilja G, et al. Hypothermia versus Normothermia after Out-of-Hospital Cardiac Arrest. N Engl J Med 2021;384:2283-94. [Crossref] [PubMed]
- Knaus WA, Draper EA, Wagner DP, et al. APACHE II: a severity of disease classification system. Crit Care Med 1985;13:818-29. [Crossref] [PubMed]
- Rittenberger JC, Tisherman SA, Holm MB, et al. An early, novel illness severity score to predict outcome after cardiac arrest. Resuscitation 2011;82:1399-404. [Crossref] [PubMed]
- Wijdicks EF, Bamlet WR, Maramattom BV, et al. Validation of a new coma scale: The FOUR score. Ann Neurol 2005;58:585-93. [Crossref] [PubMed]
- Vincent JL, Moreno R, Takala J, et al. The SOFA (Sepsis-related Organ Failure Assessment) score to describe organ dysfunction/failure. On behalf of the Working Group on Sepsis-Related Problems of the European Society of Intensive Care Medicine. Intensive Care Med 1996;22:707-10. [Crossref] [PubMed]
- Hajian-Tilaki K. Sample size estimation in diagnostic test studies of biomedical informatics. J Biomed Inform 2014;48:193-204. [Crossref] [PubMed]
- Cardona M, Lewis ET, Kristensen MR, et al. Predictive validity of the CriSTAL tool for short-term mortality in older people presenting at Emergency Departments: a prospective study. Eur Geriatr Med 2018;9:891-901. [Crossref] [PubMed]
- Fischer JE, Bachmann LM, Jaeschke R. A readers' guide to the interpretation of diagnostic test properties: clinical example of sepsis. Intensive Care Med 2003;29:1043-51. [Crossref] [PubMed]
- Christodoulou E, van Smeden M, Edlinger M, et al. Adaptive sample size determination for the development of clinical prediction models. Diagn Progn Res 2021;5:6. [Crossref] [PubMed]
- Vardon-Bounes F, Gratacap MP, Groyer S, et al. Kinetics of mean platelet volume predicts mortality in patients with septic shock. PLoS One 2019;14:e0223553. [Crossref] [PubMed]
- Adrie C, Laurent I, Monchi M, et al. Postresuscitation disease after cardiac arrest: a sepsis-like syndrome? Curr Opin Crit Care 2004;10:208-12. [Crossref] [PubMed]
- Polderman KH, Girbes AR, Thijs LG, et al. Accuracy and reliability of APACHE II scoring in two intensive care units Problems and pitfalls in the use of APACHE II and suggestions for improvement. Anaesthesia 2001;56:47-50. [Crossref] [PubMed]
- Zeng DX. Usefulness of mean platelet volume as a marker for clinical outcomes after out-of-hospital cardiac arrest J Thromb Haemost 2017;15:197-8. comment. [Crossref] [PubMed]
- Phelps R, Dumas F, Maynard C, et al. Cerebral Performance Category and long-term prognosis following out-of-hospital cardiac arrest. Crit Care Med 2013;41:1252-7. [Crossref] [PubMed]
Cite this article as: Kongratanapasert T, Vattanavanit V. Mean platelet volume for prognostication after out-of-hospital cardiac arrest: a retrospective cohort analysis. J Emerg Crit Care Med 2024;8:8.